What collection methodology to analyze Facebook posts?
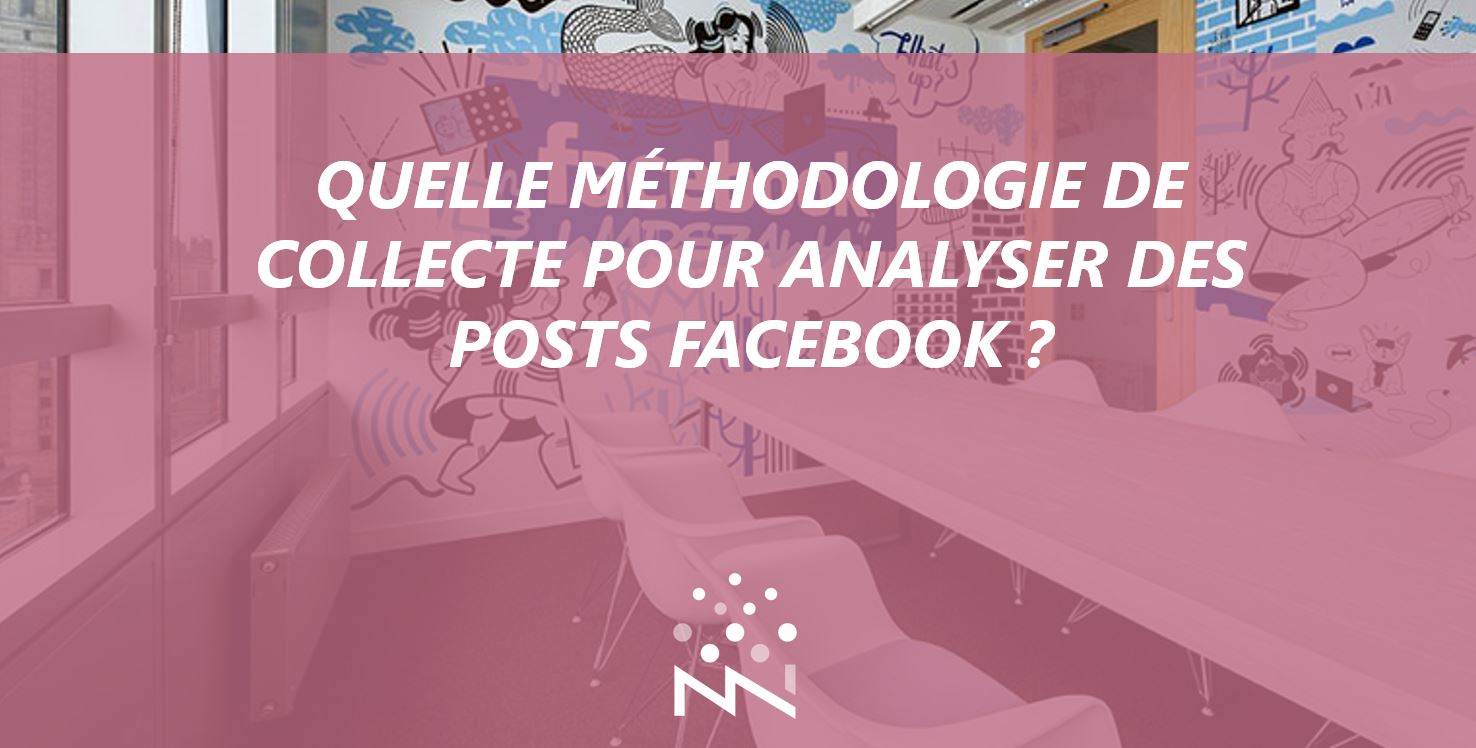
In recent years, platforms have restricted access to their data for multiple reasons: optimizing infrastructures, security, respecting the confidentiality of private data or promoting their business models. From a research perspective, this limited access to data raises numerous methodological questions for studies that rely on corpora made up of social media publications. First of all, Facebook allows access to data that it agrees to share with the market ; these are in particular the publications of so-called “public” pages that represent only a minority of the platform's activity and its interactions between users. All publications on user profiles and exchanges via messaging tools, that is to say the majority of interactions — and probably the most interesting to analyze for brands seeking to understand the behaviors of their consumers, are hidden. For several years, practitioners have been using Facebook's Graph API to collect historical data on pages. It is a tool acclaimed by brands, most often through SaaS solutions, to assess their presence on the network, question their investments or produce marketing knowledge. However, Facebook also decides how to access the data on its platform (quota, type of possible requests...) thus shaping methodologies and tools, not according to the data necessary and useful for market players but, rather, according to what is possible and authorized to do. These limitations highlight the weakness of the tools available for marketing knowledge on Facebook; this is how social listening players focus their offers on the analysis of other platforms. In this universe of possibilities, brands have understood that the data captured on platforms moves away from the representativeness regime of traditional studies, they have built analysis methodologies that rely essentially on the comprehensiveness of data sets. However, Facebook also limits access to data by selecting — without us really knowing according to what criteria — the most relevant publications.. In other words, the Facebook Graph API does not always return all the publications made by a page and, at the same time, the majority of users of these tools probably rely on truncated data sets...
We illustrate this with a common use case within communication or marketing departments who want to conduct a benchmark or report by capturing posts from Facebook pages that they are not administrators of, over a past period of time. We selected 16 Facebook pages from French-speaking media considered active contributors, publishing frequently with some regularity throughout the year. We aim to capture the comprehensiveness of their publications made in February by adopting three collection strategies:
- A common scenario : the tool makes a single request per page for the entire period. Our collection is launched on 1Er May to retrieve historical data from the 1Er to February 28. It is this method that is most frequently used by SaaS tools.
- A scenario that consumes more API requests : this time, the tool makes one request per page and per day. The collection is launched on 1Er May and we retrieve the data for February 28, then February 27, then 26, etc. for each of our media backwards.
- A scenario designed by The Metrics Factory : we recover data as we go along, day after day. Data capture starts on 1Er February at midnight and we retrieve the data from the previous day every day. In short, we are capturing historical data, but over a recent period.
The data access limits mentioned above are unequivocal. Our first two scenarios returned 3677 and 3672 posts respectively, while the methodology designed by The Metrics Factory captured 22,011 posts, or 6x more data collected with this method ! The data captured by the first two methods is nearly identical, and it's hard to understand why Facebook doesn't return exactly the same posts. However, scenario No. 2 is clearly less efficient because it consumes more API requests (16 pages x 28 days = 448 requests at least), which implies longer processing times (~1h30 vs 3min) in order to respect the quotas imposed by Facebook (200 requests per hour).

The data returned by the methodology designed by The Metrics Factory draws a completely different pattern than that of the other two approaches. The publications made by the media are regular, and have a high seasonality with peaks of activity on working days and troughs on weekends. Conversely, the data collected by the usual method reveals the selection of posts made by Facebook: around 35% of posts are returned in the first few days, then the volume fades to around 5% for older posts.

Even worse, The most active pages are represented in a minority way in the results of the first collection. With The Metrics Factory method, we were able to retrieve 2328 posts published by La Provence between 1Er and on February 28 while with the usual method, no posts were returned. It therefore becomes impossible to retrieve historical data for pages beyond a certain time... This is what we can see in the graph below: Konbini publishes relatively little compared to 20 minutes, we were able to capture posts for the entire period. On the other hand, for 20 minutes, the API no longer returns information after 9 days.
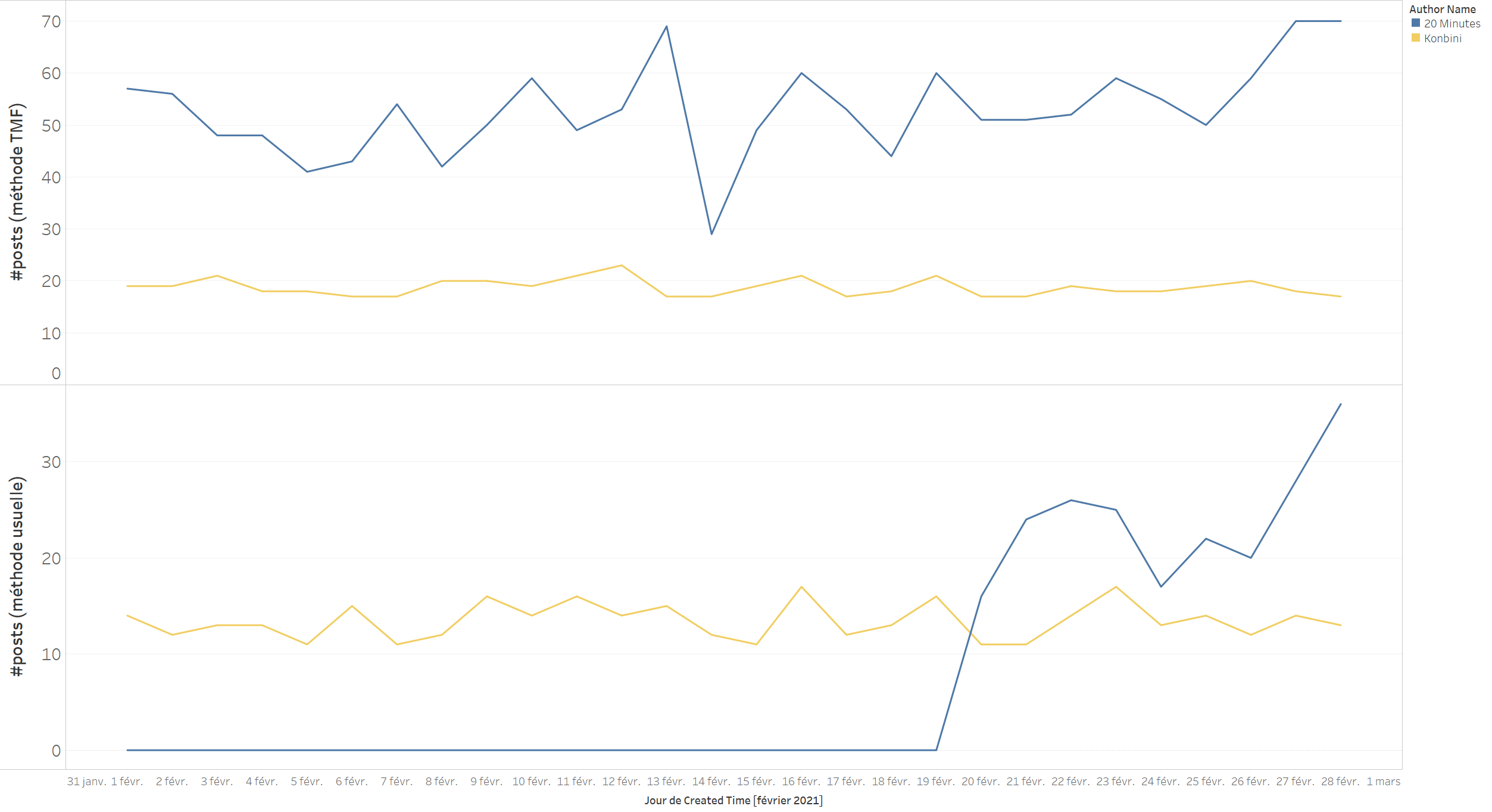
The most surprising is the method of selecting publications: The API does not return the posts with the most commitments. The usual collection method (scenario 1) only returns 28% of the total commitments over the period. For example, this BFM TV post (115k commitments — 3)E post with the most commitments over the period), these Images of a minister arousing controversy (60k commitments) or this more recent news (11k commitments) were not captured using the usual method.
The points to remember:
- Platforms limit access to their data, but also the modalities of this access.
- This limited access raises methodological questions and shapes market tools according to the conditions of access to data.
- Data completeness is never guaranteed, as platforms select what can be collected.
- Without a trusted third party, the quality of the data is not guaranteed either: the data collected comes directly from the platform, without a certificate of their integrity.
- The interpretation of the data is all the more difficult since the criteria for selecting the returned data are not known.